How Speech Emotion Detection (SED) Boosts User Experience?
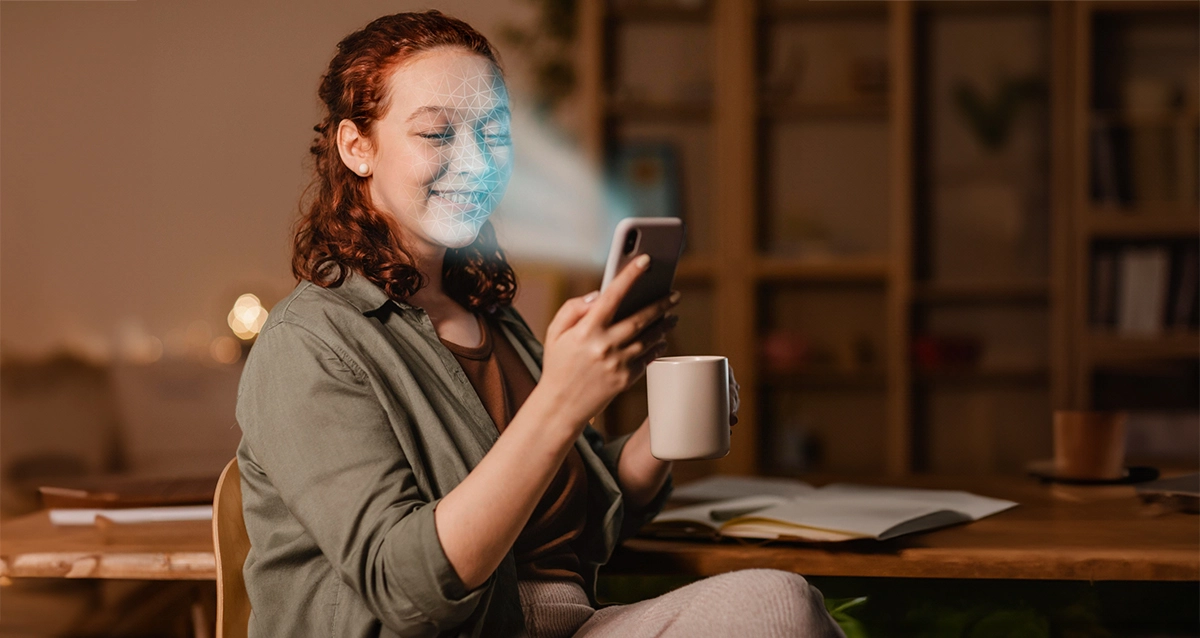
5 min read
Today, non-verbal communication such as tone, pitch, speaking rate, and choice of words reveal our attitude and state of mind as much as the intent of the words we say. However, new AI and ML technologies now allow systems to identify emotions from the voice in an instant accurately. This new frontier of speech emotion technology is known as speech emotion recognition/detection (SER/SED).
So, join us in this exploration of how SED plays a role in the user experience and how it is a stepping stone to new possibilities and better interactions.
Defining Speech Emotion Detection/Recognition
Speech emotion detection/recognition is a process where the emotion that a speaker is in is determined by the sounds produced. An example application can easily be seen in call centers where SER could be used with the uses of artificial intelligence to determine when a caller is frustrated and connect them to agents who are trained to handle such callers to ensure maximum customer satisfaction.
For example, if the customer begins yelling or speaking quickly, the speech system may identify the signs of aggression or stress and forward the call to a manager trained to deal with an irate client.
A few benefits include:
- Enhanced customer support.
- Improved virtual assistant interactions.
- Individualized interactions within applications and games.
- Early disease detection and better mental health management.
- Better ways of reaching the customers and concentrating on them.
What Components make up Speech Emotion Recognition?
The common mode of operation of the SER is that it takes speech audio as the input and then provides the output which is the emotional state of the speaker. Here are some key components of emotion recognition in speech:
1. Feature Extraction
In the first stage of speech emotion recognition, the audio signal is processed so that one can derive the pitch, intensity, speaking rate, spectrum characteristics, and so forth. These characteristics are related to different emotions.
2. Emotional Database
Machine learning models are trained on a large emotional speech database to achieve high performance. The database includes 10×10=100 audio samples labeled with basic emotions, such as happy, sad, angry, neutral, etc.
3. Machine Learning Models
Such types of classifiers as support vector machines (SVM), artificial neural networks (ANN), random forests, and others are applied to the database of emotional speech samples. During the training, the models adapt to categorizing emotions based on the acoustic features.
4. Emotion Classifier
When employing SER, the feature extraction component processes test speech samples and extracts various features related to the acoustic characteristics of the samples. These features are passed through a trained machine learning model which then determines the emotion recognition in speech based on the learned patterns.
5. Output Emotion
In the end, the identified emotions such as happy, angry, sad, etc. are available at the output of the speech emotion recognition system. Other systems are even capable of identifying higher levels of emotions together with the level of emotion as well.
Hence, it can be seen that the greater the size of the database used in the training of the models for the classification of emotional speeches, the better the performance of the SER. It also evolves as the AI & ML services advance in their models.
How do AI and Machine Learning Relate to SED?
With the help of approaches such as advanced algorithms and neural networks, machine learning services can turn the initial signals into emotions and, thus, contribute to the development of new approaches to the user interface and experience. Examples include:
Support Vector Machines (SVMs)
SVMs are used in the field of machine learning where data is provided, and patterns are looked for together with classification and regression. In speech emotion recognition, SVMs can be trained from an audio database, labeled with one of the emotional tags to classify new speech samples into various emotional categories.
Random Forests
Random forests are machine learning techniques that work by using multiple decision trees resulting in the class that is the most frequently predicted by the individual trees. In the case of emotion recognition in speech, the features of the speech could be input into the random forest which then predicts the emotion type from the training data.
Artificial Neural Networks (ANNs)
In speech emotion recognition, ANNs accept speech audio as input where hidden layers analyze the characteristics and nuances of the audio to classify it according to the trained parameters. Other advanced ANNs such as CNNs and RNNs are also used. Just like any other complex ANNs, CNNs, and RNNs are also used.
Convolutional Neural Networks (CNNs)
CNNs are a particular kind of neural network, used with the data which has a grid-like structure, such as images. Regarding spectrogram data derived from the speech, CNNs assist in properly assessing the texture and features in comparison with the taught labels of emotions.
Recurrent Neural Networks (RNNs)
For sequential data, RNNs are beneficial for SED tasks, including LSTM. This is because their repeating hidden state allows for the modeling of other long-range contextual emotion dependencies in speech. The mechanisms of memory and gating prevent the vanishing gradient problem during the training process.
Top Five Use Cases Where SED Boosts User Experience
Consider a situation in which machines can comprehend and react to people’s feelings in the same manner as another individual. This is gradually becoming a reality through SED commonly referred to as Speech Emotion Detection. Thus, the following is the list of machine learning use cases where SED is having a noticeable effect.
1. Call Centre Customer Service
If a caller is upset, angry, or distressed, it will help the customer care provider to understand and address their concerns. For instance, if a customer seems very angry while seeking redressal for any concern, the agent can empathize and assure him/her that rectification of the problem is the main goal.
2. Clinical Diagnosis
The vocal intonations of a patient that are indicative of anxiety, sadness, or hopelessness may indicate the need for a mental health check-up by a healthcare professional. For instance, machine learning application in healthcare – SED may help to shine a light on whether a patient is concealing suicidal ideation.
3. Instruction
Teachers can identify when a learner is bored, confused, or disengaged during remote learning and adapt their teaching strategies. For instance, if a teacher and a student are on a video call, and the student looks disinterested, then the teacher will incorporate more discussions into the lecture.
4. Transportation/Auto
If the driver’s voice was considered drowsy, distracted, or angry, it could lead to safety mechanisms in cars or trucks being enacted. For instance, imagine you’re traveling in a cab whose driver is napping. In that case, identifying fatigue would result in the emission of a seat vibration signal to wake a dozing driver.
5. Smart Home Devices
Perhaps, knowing that a user is annoyed when requesting help with a chore would help AI voice assistants like chatbots to respond more effectively. For instance, a question containing anger or disbelief about the directions could be met with a softer response from a navigation assistant.
Future Prospects for Speech Emotion Detection Theory
Speech emotion detection is a recent & promising field of study that deals with the real-time identification of emotions from voice data. Some of the possible future directions for further development of this field are as follows:
- Emotion recognition within videos and other physiological indicators.
- Model generalization for machine learning applications and populations.
- Development of new advanced approaches to learn from less labeled data.
- Studies on combining audio, video, and textual data for emotion recognition.
- Deep learning and neural networks for learning feature representations.
There is a lot of opportunity in speech emotion detection for developing realistic human-like communication. With machines, provided major barriers of practical use such as robustness, interpretability, and fairness are addressed in future cross-disciplinary studies.
Conclusion
Speech emotion recognition presents an immense opportunity for improvement in the way humans interact with machines. It also means that all the companies that will implement this technology will have a competitive advantage as soon as emotional intelligence. It becomes a standard in all interfaces used by customers. Therefore, by properly identifying and then addressing emotions in a given situation, we can develop a smarter and kinder technological world.
Published: August 26th, 2024