How Deep Learning will Impact in Healthcare
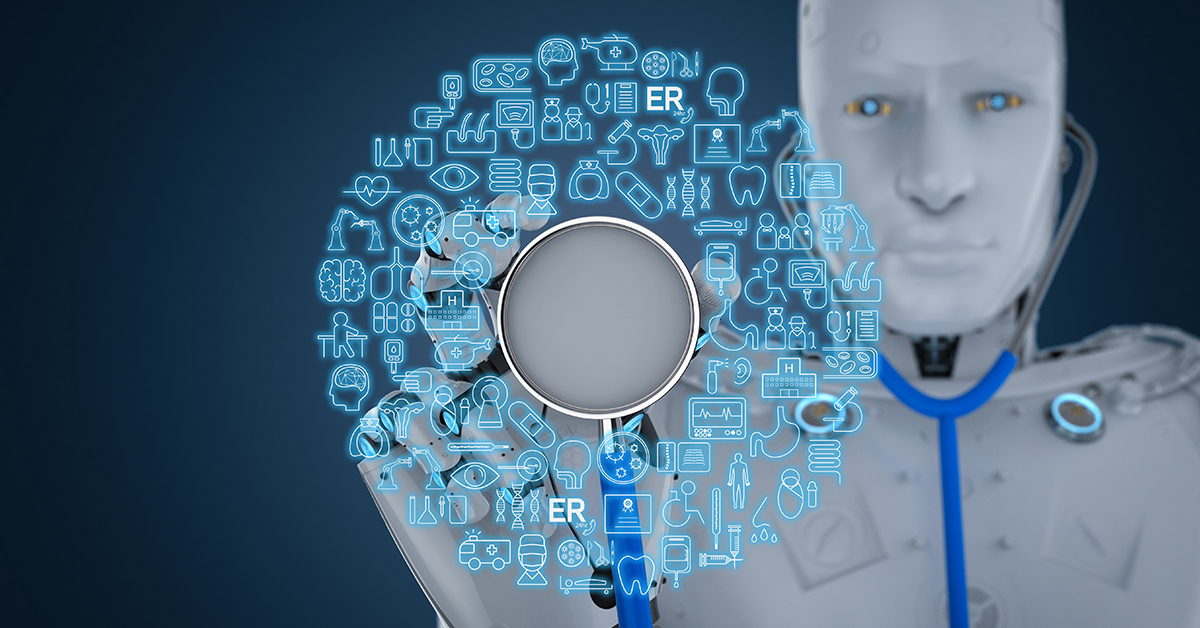
4 min read
Introduction
With the rise of lifestyle-related diseases and the rapidly growing global population, our modern healthcare system is currently dealing with enormous challenges, where the incorporation of deep learning holds promising solutions.
The good news is that healthcare could become more affordable, efficient, individualized, and equitable. By using AI ML DL to develop intelligent processes and workflows.
The fourth industrial revolution’s profound changes are expected to have the greatest impact on the healthcare industry. In order to efficiently and effectively choose between vendor products or hire the right data science staff to develop algorithms in-house.
Healthcare organizations should be confident that they have a thorough understanding of the various flavors of artificial intelligence services and how they can apply to specific use cases.
Starting with deep learning is wise. This area of artificial intelligence has quickly revolutionized the healthcare industry. By enabling data analysis at a speed and accuracy that have never been possible.
However, what precisely is deep learning? How does it differ from other machine learning techniques? How can healthcare organizations use deep learning methods to address some of the most urgent issues in patient care?
Where Does Deep Learning Apply In The Healthcare Sector?
Deep learning-related news stories in the industry frequently involve pre-commercial research projects or small-scale pilot tests.
Deep learning is, however, increasingly being incorporated into cutting-edge tools with significant clinical applications. Innovative patient-facing software and a few surprisingly old methods for enhancing. The user experience of health IT is one of the most promising use cases.
Analytics and Diagnostics for Imaging
Convolutional neural networks (CNNs), a specific kind of deep learning. They are especially effective at analyzing images, such as x-rays or MRI scan results.
Experts in computer science at Stanford University claim that CNNs will be built with the intention of processing images. Which enables the networks to operate more effectively and handle larger images.
As a result, when it comes to recognizing crucial details in diagnostic imaging studies. Some CNNs are getting close to—or even eclipsing—the precision of human diagnosticians. Deep learning tools are not only quick but also extremely accurate. Because of their accuracy, these simulated images are used to train future deep learning models to identify clinical findings.
According to the team, “medical imaging data sets are frequently unbalanced as pathologic findings are typically uncommon. Which presents tough problems for deep learning model training. We suggest a technique for creating fake abnormal MRI images with brain tumors using generative adversarial network training.
” This provides a source of diverse data that is automatable, affordable, and is used to supplement the training set.
To routinely alter a tumor’s size, location, or placement, or to insert it into an otherwise healthy brain.
Such a plan could significantly lessen one of AI’s biggest problems. A dearth of trustworthy, shareable, large-scale datasets for machine learning model training and validation.
The Processing of Natural Language
Many of the NLP tools that have gained popularity in the healthcare sector, for transcription of speech to text and dictation of documentation are already based on deep learning and neural networks.
By “grouping” related words together and mapping them in relation to one another. Neural networks—which create for classification—can recognize specific linguistic or grammatical elements.
Another issue is finding sufficient high-quality data sources to properly train models. Models may not be able to accurately assess a wide range of patients in real-world settings.
Even so, a few forward-thinking programmers are figuring out ways to get past the obstacles in the way of deep learning. Which is the most promising route to reliable free-text analytics.
Drug Development and Precision Medicine
Deep learning developers are interested in drug discovery and precision medicine. To uncover previously undiscovered relationships between genes, medications, and physical environments. Both tasks involve processing truly enormous volumes of genomic, clinical, and population-level data.
Deep learning is a great method for researchers and pharmaceutical stakeholders. Those who want to find novel patterns in these largely unexplored data sets, particularly since many researchers in precision medicine are still unsure of what patterns they should be looking for.
Molecular modeling and predictive analytics should help researchers learn more about how and why particular cancers develop in particular patients. According to the two agencies, deep learning technologies will quicken the data analysis process by cutting the processing time for crucial elements from weeks or months to just a few hours.
The private sector is planning similar efforts to show how effective deep learning can be in precision medicine.
With the help of molecular modeling and predictive analytics, it is hoped that new knowledge will be gained about the causes and progression of specific cancers in particular patients.
The tool provides human clinicians with a thorough explanation of why it recommends a particular course of action, helping to build trust and giving providers confidence in their own judgment when potentially defying the algorithm.
What Does The Future of Deep Learning in Healthcare Look Like?
These pilots and projects are intriguing. As they may be, they are just the beginning of deep learning’s application to healthcare analytics.
Deep learning is generating excitement and interest across industries, catching the attention of policymakers, business owners, healthcare professionals, and even patients.
One organization, the Office of the National Coordinator (ONC), has particularly high expectations for deep learning and has already praised some web development companies for their outstanding accomplishments.
The agency recently published a report on the state of AI in healthcare. It noted that some deep learning algorithms have already yielded “transformational” results.
There have been significant examples of the potential use of deep learning-based artificial intelligence techniques in medical diagnosis.
Applications of deep learning algorithms in clinical settings offer the potential to consistently deliver high-quality results. Where good training sets represent the highest levels of medical expertise.
Early successes in diabetic retinal screenings and the classification of skin cancer were highlights in the report as two instances where deep learning is already be altering the status quo. Given that deep learning already has an advantage in many high-value applications on the clinical side, imaging analytics is likely to be the focus for the foreseeable future.
Conclusion
An era of improved diagnostics, personalized treatments, effective drug development, and preventive care is about to begin thanks to deep learning’s potential to revolutionize the healthcare industry.
Healthcare providers can make more informed decisions that improve patient outcomes and contribute to a healthier society by utilizing AI to analyze massive amounts of data and spot complex patterns. To ensure that the application of deep learning in healthcare is safe, effective, and patient-centered as technology develops. It is crucial to strike a balance between embracing innovation and upholding ethical principles.
Published: July 26th, 2023